AI-powered data analytics tool for predicting reservoir properties
EarthNET AI Seismic properties lets you propagate knowledge from the well to seismic scale to predict reservoir properties from elastic properties generated from well data, either as a function of 3D partial stacks, or as a function of partial-stack cubes.
- MODEL TRAINING
- SCALING
- SEISMIC WELL-TIE
- MODEL QC
- UNCERTAINTY CUBE
- ACTIVE LEARNING
Model training
With EarthNET Seismic properties, you can train models to predict many different properties from seismic, including vp, vs, vp/vs, density, acoustic impedance, lithology, porosity, vclay, permeability, water saturation, and more. The property you want to predict needs to be represented in the form of a well log, and there should be a discernable relationship between the property and the seismic data that can be learned during the model training process.
You get access to a large number of deep learning model architectures in EarthNET including neural networks such as Unet’s and transformers. We ensure that we keep up with the latest advancements in ML architecture and provide you with the most advanced and accurate models.
Scaling methods
Since seismic data has a coarse sampling interval, whereas well data has a fine sampling interval, you need to scale the well data to the seismic data.
The scaling functionality in EarthNET ensures that the detail level between the 3D volume features and the well log responses are comparable.
Seismic well-tie
Seismic data is recorded in time (TWT) whereas well data is recorded in depth (MD). In order to use well data in combination with seismic data, we need to ensure that both datasets are aligned. EarthNETs 'seismic well tie tool lets you quantify this time-depth relationship.
Model QC
Review and compare model results and performance metrics for multiple models to identify the models that perform best for your tasks.
All your trained ML models will be stored in your model library, along with all the metadata that describes the training data used, the model architecture, parameters, and results. With full information about each model, you can quickly identify potential causes for suboptimal performance, mitigate them and re-train the model.
Uncertainty cube
The prediction of properties from seismic is a process that provides non-unique solutions, meaning that the results are always uncertain. It is therefore crucial to quantify this uncertainty, and EarthNET provides the tools to do so.
By using our uncertainty cube functionality, you can obtain a standard deviation volume that describes the uncertainty in your predicted property. This enables you to assess where the uncertainty is high and where it is low, which is critical when providing the basis for decision-making.
Active learning
The infrastructure in EarthNET facilitates active learning to ensure that you have a set of models that improve over time with minimal effort.
EarthNET supports active learning workflows through quantifying model performance, improving input data through data cleaning and labeling, retraining models using the improved input data, and tracking model performance over time.
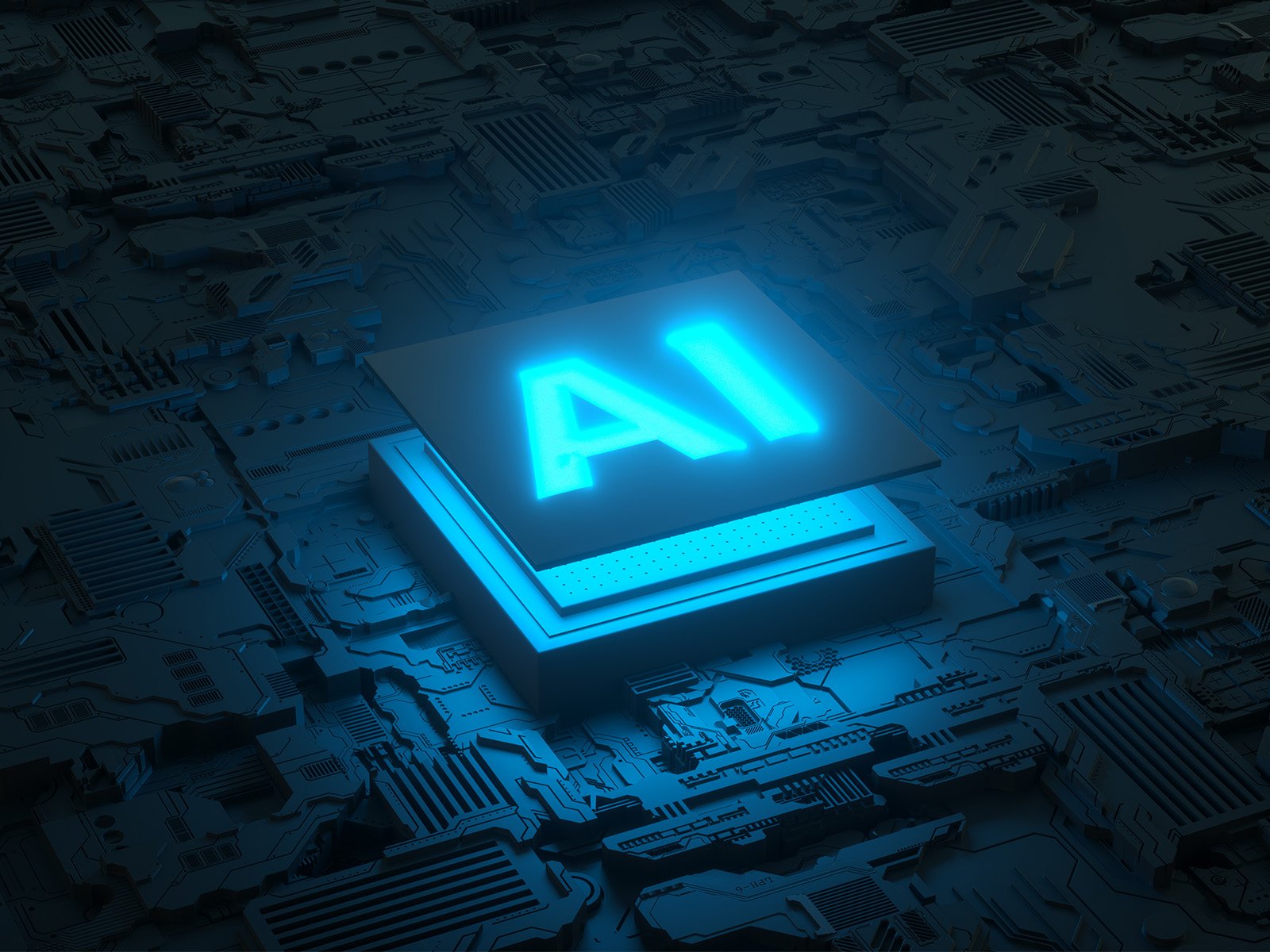
Revolutionise seismic inversion workflows with AI
Quantifying the reservoir's properties is essential for populating geocellular models for volumetric calculations and flow simulation studies, and for identifying exploration opportunities and potential carbon storage sites. Traditionally, predicting reservoir properties is done by geophysicists through seismic inversion and rock physics. This multi-step process is both time-consuming and costly, and demands the expertise of highly skilled individuals.
EarthNET AI Seismic properties has revolutionised the seismic property prediction process into one seamless workflow using AI. You can now create a 3D property cube in a matter of weeks, which would be impossible using the traditional seismic inversion workflow.
Propagate knowledge from well to seismic scale
Models can be trained to predict any desired property that is available as well logs, including acoustic impedance, Vp/Vs. density, lithology, porosity, Vclay, and water saturation (Sw).
Select seismic survey, partial-stacks and velocity volume to be used as input features in the AI model
Select the wells you want to train on, and the well log that best represents your target property.
Use scaling methods to ensure that the detail level between the 3D volume features and the well log responses are comparable.
Use the seismic well-tie functionality in EarthNET or import well-ties from your preferred software to ensure precise feature-to-label alignment.
Choose between a range of fully customisable Deep Neural Network architectures to train your property prediction model.
Train the model on the wells and seismic you have selected using high-performance cloud computing.
Evaluate model according to metrics and visual displays. Review the model's predictions against labels and perform a geological sense check.
Use the model to make predictions on the complete data set and retrieve the full 3D property volume.
Obtain a standard deviation volume that describes the uncertainty in the predicted property